Sep 30, · If you faced any problems during the data collection or analysis phases, use the methodology section to talk about what you did to address these issues and minimise the impact. Final Thoughts Whether you are completing a PhD or master's degree, writing your thesis or dissertation methodology is often considered to be the most difficult and time The prior research section in particular must be more comprehensive, although you may certainly summarize your report of prior research if there is a great deal of it. Your actual dissertation will be the obvious place to go into more detail. The research approach or methodology section (5) should be explained explicitly Feb 25, · In quantitative research, your analysis will be based on numbers. In the methods section you might include: How you prepared the data before analyzing it (e.g. checking for missing data, removing outliers, transforming variables) Which software you
How to Write a Research Methodology in Four Steps
Try out PMC Labs and tell us what you think. Learn More. Gene set enrichment GSE analysis is a popular framework for condensing information from gene expression profiles into a pathway or signature summary. The strengths of this approach over single gene analysis include noise and dimension reduction, data analysis section of dissertation, as well as greater biological interpretability.
As molecular profiling experiments move beyond simple case-control studies, robust and flexible GSE methodologies are needed that can model pathway activity within highly heterogeneous data sets. To address this challenge, we introduce Gene Set Variation Analysis GSVAa GSE method that estimates variation of pathway activity over a sample population in an unsupervised manner. We demonstrate the robustness of GSVA in a comparison with current state of the art sample-wise enrichment methods.
Further, we provide examples of its utility in differential pathway activity and survival analysis. Lastly, we show how GSVA works analogously with data from both microarray and RNA-seq experiments. GSVA provides increased power to detect subtle pathway activity changes over a sample population in comparison to corresponding methods. While GSE methods are generally regarded as end points of a bioinformatic analysis, GSVA constitutes a starting point to build pathway-centric models of biology.
Moreover, GSVA contributes to the current need of GSE methods for RNA-seq data. The ability to measure mRNA abundance at a genomic scale has led to many efforts to catalog the diverse molecular patterns underlying biological processes. To facilitate the interpretation and organization of long lists of genes resulting from microarray experiments, gene set enrichment GSE methods have been introduced.
They systematically measure and annotate molecular profiles that are inherently noisy and difficult to interpret, data analysis section of dissertation. GSE analyses begin by obtaining a ranked gene list, typically derived from a microarray experiment that studies gene expression changes between two groups.
The genes are then mapped into predefined gene sets and their gene expression statistic is summarized into a single enrichment score for each gene set. A significant benefit of these pathway-based methods is interpretability: gene function is collectively exerted and may vary by environmental stimuli, genetic modifications, or disease state. Thus, organizing genes into gene sets provides a more intuitive and stable context for assessing biological activity.
Many methodological variations of GSE methods have been proposed [ 1 - 6 ], including non-parametric enrichment statistics [ 47 ], battery testing [ 8 - 10 ], and focused gene set testing [ 11112 ]. Battery testing methods aim at identifying gene sets standing out from a large collection of annotated pathways and gene signatures. Focused gene set testing methods try to carefully evaluate a few gene sets that are relevant to the experiment being analyzed [ 12 ]. GSE methods have been successfully applied in many experimental conditions to interpret the pathway architecture of biological states including cancer [ 1314 ], metabolic disease [ 15 ], and development [ 16 ].
For a recent review on GSE methods the reader may consult [ 17 ]. An important distinction among many of the GSE methods is the definition of the null hypothesis that is tested [ 18 ].
The null hypothesis of a competitive test declares that there are no differences between genes inside and outside the gene set e. A self-contained test defines its null hypothesis only in terms of the genes inside the gene set being tested e. More concretely, for a self-contained test on a gene set, the differential expression of just one of its genes allows one to reject the null hypothesis of no differential expression for that gene set.
It follows, that self-contained tests provide higher power than competitive tests to detect subtle changes of expression in a gene set. But they may not be useful to single out a few gene sets in a battery testing setting because of the potentially large number of reported results. Finally, many GSE methods assume two classes e. govan ambitious project with the goal to identify the molecular determinants of multiple cancer types.
In contrast to case-control studies with small sample sizes, the TCGA project has large patient cohorts with multiple phenotypes, structured with hierarchical, multi-class, and censored data. Hence, GSE methods are needed that can assess pathway variation across large, data analysis section of dissertation, heterogeneous populations with complex phenotypic traits.
To address these challenges, we present a non-parametric, unsupervised method called Gene Set Variation Analysis GSVA. GSVA calculates sample-wise gene set enrichment scores as a function of genes inside and data analysis section of dissertation the gene set, analogously to a competitive gene set test, data analysis section of dissertation. Further, it estimates variation of gene set enrichment over the samples independently of any class label.
Conceptually, this methodology can be understood as a change in coordinate systems for gene expression data, from genes to gene sets. This transformation facilitates post-hoc construction of pathway-centric models, such as differential pathway activity identification or survival prediction.
Further, we demonstrate the flexibility of GSVA by applying it to RNA-seq data analysis section of dissertation. Let γ k be the number of genes in γ k. GSVA methods outline. The input for the GSVA algorithm are a gene expression matrix in the form of log2 microarray expression values or RNA-seq counts and a database of gene sets. Kernel estimation of the cumulative density function kcdf. The two plots show two simulated expression profiles mimicking 6 samples from microarray and RNA-seq data.
The x -axis corresponds to expression values where each gene is lowly expressed in the four samples with lower values and highly expressed in the other two. The scale of the kcdf is on the left y -axis and the scale of the Gaussian and Poisson kernels is on the right y -axis.
The expression-level statistic is rank ordered for each sample. For every gene set, the Kolmogorov-Smirnov-like rank statistic is calculated. The plot illustrates a gene set consisting of 3 genes out of a total number of 10 with the sample-wise calculation of genes inside and outside of the gene set. The GSVA enrichment score is either the maximum deviation from zero top or the difference between the two sums bottom. The two plots show two simulations of the resulting scores under the null hypothesis of no gene expression change see data analysis section of dissertation text.
The output of the algorithm is a matrix containing pathway enrichment scores for each gene set and sample. GSVA starts by evaluating whether a gene i is highly or lowly expressed in sample j in the context of the sample population distribution. Probe effects can alter hybridization intensities in microarray data such that expression values can greatly differ between two non-expressed genes [ 23 ], data analysis section of dissertation.
Analogous gene-specific biases, such as GC content or gene length have been described in RNA-seq data [ 24 ]. To bring distinct expression profiles to a common scale, an expression-level statistic is calculated as follows. In the case of microarray data, a Gaussian kernel [ 25 ], pg. In the case of RNA-seq data, a discrete Poisson kernel [ 26 ] is employed:. The following step condenses expression-level statistics into gene sets by calculating sample-wise enrichment scores.
This is done to up-weight the two tails of the rank distribution when computing the final enrichment score. Conceptually, Eq. We offer two approaches for turning the KS like random walk statistic into an enrichment statistic ES also called GSVA scorethe classical maximum deviation method [ 42728 ] and a normalized ES.
The first ES is the maximum deviation from zero of the random walk of the j -th sample with respect to the k -th gene set:. This is an intrinsic property of the KS like random walk, data analysis section of dissertation generates non-zero maximum deviations under the null distribution.
In GSEA [ 4 ] it is also observed that the empirical null distribution obtained by permuting sample labels is bimodal and, for this reason, significance is determined independently using the positive and negative sides of the null distribution. In our case, we would like to provide a standard Gaussian distribution of enrichment scores under the null hypothesis of no change in pathway activity throughout the sample population.
This statistic may be compared to the Kuiper test statistic [ data analysis section of dissertation ], which sums the maximum and minimum deviations to make the test statistic more sensitive in the tails. There is a clear biological interpretation of this statistic, it emphasizes genes in pathways that are concordantly activated in one direction only, either over-expressed or under-expressed relative to the overall population. For pathways containing genes strongly acting in both directions, the deviations will cancel each other out and show little or no enrichment.
Because this statistic is unimodal and approximately normal as observed via simulation, see belowdata analysis section of dissertation, downstream analyses which may impose distributional assumptions on the data are thus possible. In such circumstances, the statistic defined by Eq. One hundred gene sets are uniformly sampled at random from the p genes with sizes ranging from 10 to genes. Using these two inputs, we calculate the maximum deviation ES and the normalized ES.
Although the GSVA algorithm itself does not evaluate statistical significance for the enrichment of gene sets, significance with respect to a data analysis section of dissertation can be easily evaluated using conventional statistical models.
Likewise, false discovery rates can be estimated by permuting the sample labels Methods. We make no general prescription for thresholds of significance or false discovery, as these choices are highly context dependent and may vary according to each experiment. Examples of these techniques are provided in the following section. Methods for gene set enrichment can be generally partitioned according to the criteria of supervised vs unsupervised, and population vs single sample assessments.
Most GSE methods, such as GSEA [ 4 ], are supervised and population based, in that they compute an enrichment score per gene set to describe the entire data set, modeled on a phenotype discrete, such as case-control, or continuous. The simplest of this genre is described by Tian et al. case vs control of a set of genes, compared to those genes not in the gene set. One of the major drawbacks of this method is that gene correlations are not taken into account, which might lead to an increased number of false-positive gene sets with respect to GSEA [ 30 ].
Many other supervised, population based approaches have also been described [ 12172031 - 34 ]. A supervised, single sample based approach was introduced in the ASSESS method [ 27 ], data analysis section of dissertation.
This method is well-suited for assessing gene set variation across a dichotomous phenotype. GSVA also utilizes density estimates for evaluating sample-wise enrichment, but by omitting phenotypic information, data analysis section of dissertation, it enables more general downstream analyses and therefore broader applications. Three unsupervised, single sample enrichment methods have been developed, data analysis section of dissertation, Pathway Level analysis of Gene Expression PLAGEsingle sample GSEA ssGSEA and the combined z-score [ 52235 ].
These methods compute an enrichment score for each gene set and individual sample. PLAGE standardizes each gene expression profile over the samples and then estimates the data analysis section of dissertation activity profiles for each gene set as the coefficients of the first right-singular vector of the singular value decomposition of the gene set [ 35 ], pg. The combined z-score method [ 22 ] standardizes first, as PLAGE, each gene expression profile into z-scores but the pathway data analysis section of dissertation profile is then obtained by combining the individual gene z-scores per sample [ 22 ], Figure one.
Both, PLAGE and the combined z-score are parametric and assume that gene expression profiles are jointly normally distributed, data analysis section of dissertation. The combined z-score additionally assumes that genes act independently within each gene set.
The ssGSEA method from Barbie et al. GSVA is unsupervised and yields single sample enrichment scores, data analysis section of dissertation. Therefore, we can directly compare the performance of GSVA to the combined z-score, single sample GSEA and PLAGE [ 52235 ], data analysis section of dissertation.
However, in contrast to the other methods, GSVA calculates first an expression statistic with the kernel estimation of the ECDF over the samples, data analysis section of dissertation, which should help in protecting the method against systematic gene specific effects, such as probe effects, and therewith increase its sensitivity. To verify this hypothesis we have performed the following three simulation studies.
Using this model we have generated data sets of increasing sample size and defined two gene sets formed by 30 genes each, where one gene set is differentially expressed DE and the other is not.
How to Write a Dissertation Results Section - Scribbr
, time: 3:54Principal component analysis: a review and recent developments
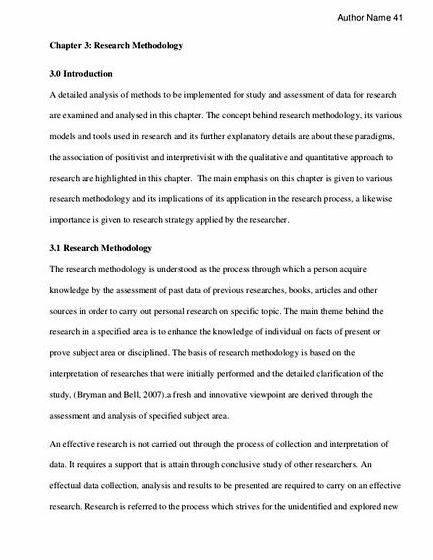
Jan 16, · Survival analysis in a TCGA ovarian cancer data set. Predictive performance in the survival analysis of a TCGA ovarian cancer microarray data set of n= samples, measured by the concordance index obtained from a 5-fold cross-validation from (A) the training data and (B) the test data. Diamonds indicate means in boxplots Apr 13, · (a) Principal component analysis as an exploratory tool for data analysis. The standard context for PCA as an exploratory data analysis tool involves a dataset with observations on p numerical variables, for each of n entities or individuals. These data values define p n-dimensional vectors x 1,,x p or, equivalently, an n×p data matrix X, whose jth column is the vector x j of observations Data quality refers to the state of qualitative or quantitative pieces of information. There are many definitions of data quality, but data is generally considered high quality if it is "fit for [its] intended uses in operations, decision making and planning". Moreover, data is deemed of high quality if it correctly represents the real-world construct to which it refers
No comments:
Post a Comment